By Sae Ra Germaine, Deputy CEO and Manager, Member & Academic Services, CAVAL (saera.germaine@caval.edu.au)
As a self-confessed conference organising addict I’ve seen my fair share of conference platforms. Before COVID there was a smattering of virtual conference platforms available and to be honest they were all terrible. There just wasn’t the need to develop something that was user-friendly and created an event “experience” that we all grew to love in the “meatspace”.
Since then… well, COVID happened. It saw this accelerated need to bring the conference space into the virtual space and the rapid growth in many cases didn’t do us many favours. We now have an over-abundance of event management platforms and I for one suffered from a severe case of choice overload.
A bit of background about me, I’m currently the Co-Chair of VALA2024 Conference, founder of the Everything Open Conference, and a “Ghost of Conferences Past” for Linux Australia. In total, for the various organisations I am linked to, I have helped in some sort of form, run 13 conferences. 4 of those were in a virtual environment with no face-to-face attendance, while 2 of those were in a hybrid form. The concept of a “Ghost of Conferences Past” is a group of those who have organised a conference prior to the current conference organising team. This group passes on knowledge, wisdom, and war stories to the current team, so they learn from victories and mistakes of conferences past.
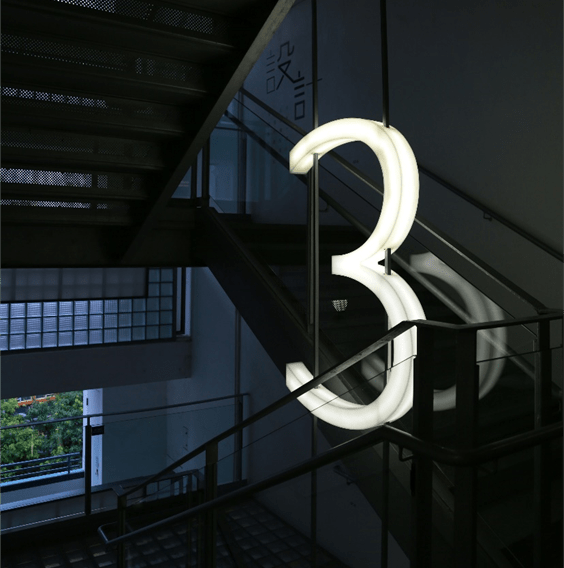
One of the greatest words of wisdom that was ever dealt out was: “You only need to deliver 3 things for a successful conference: speakers, delegates, and venue”. The venue could be the crappiest venue available, but the important piece was that people were there to learn from each other and that’s all that matters. Some of the best conferences I attended were held at a school camp venue, with post-it notes on a wall for a schedule, terrible Subway for lunch, and a whole bunch of new people to meet.
Most conferences I have run have been 100% volunteer effort. Most core teams had about 6-10 people and then about 30 volunteers on the ground during the conference. Many of these volunteers had many hats ranging from Rego Desk-ers to speaker wranglers, AV recorders, MCs, code of conduct teams, and volunteer well-being checkers. A personal plea… please don’t forget volunteer well-being checkers. This is so important! Volunteers need to be looked after too!
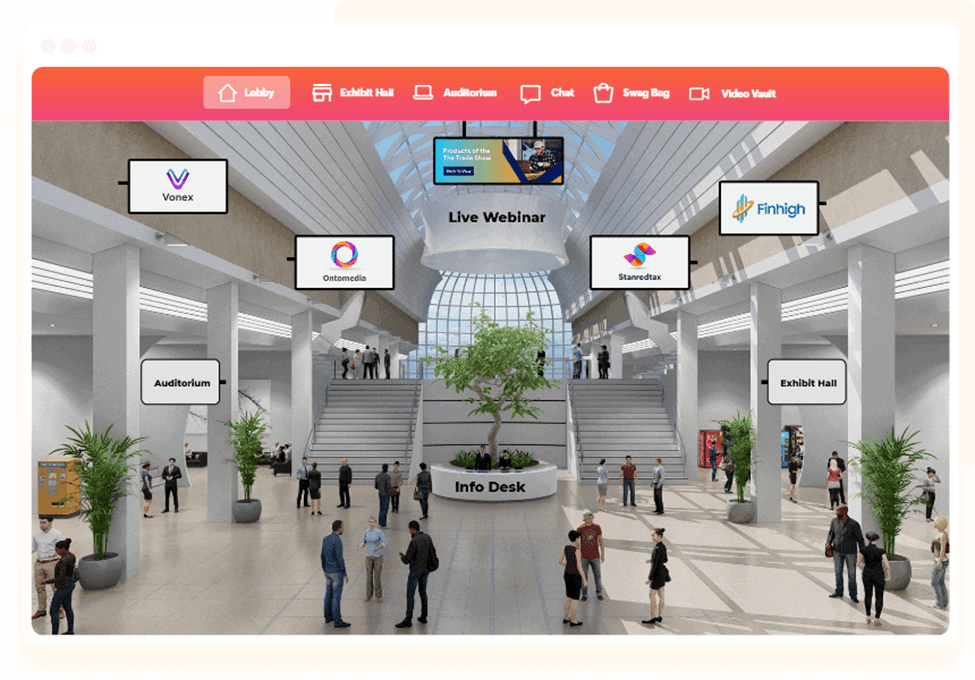
As I mentioned, COVID caused many platforms to pop-up and I’m not sure why this is but, so many platforms insisted on re-creating the “meatspace” conference experience in a virtual environment. You know what? It does not work! It creates a confusing space for people, and it makes it difficult to manage by your team.
A fancy virtual world creating experiences are very IT resource intensive. Remember, given Australia’s terrible internet situation you want to deliver a conference to reach the regions that will experience your conference with a horrible internet connection. The true benefit to running an online conference is to maximise your reach, don’t exclude those you are trying to reach by choosing a platform that a bad internet connection will struggle to deliver.
I’m not going to list and do comparisons on lots of platforms as we all will make decisions on the platform we use based on needs at the time and what would provide the best experience possible. But, what I will do is talk about 3 specific setups that I’ve found to work very well for the events that I’ve helped run. The key with all 3 is the usability of the interface – in a virtual world, the venue does matter! The first 2 setups will require a separate mechanism for managing sponsorships, vendors, and schedules/website, etc.
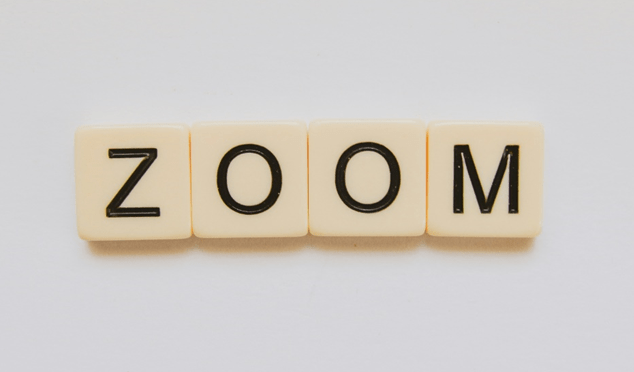
Zoom:
Love it or hate it, Zoom is one of the most common tools used across sectors. It can be downloaded or used directly within a browser. Zoom has also added an “Events” platform to help manage ticket sales and while it’s not perfect, it will get there eventually. One of the best conferences I attended was multiple zooms that I could duck in and out of and the schedule was in a simple Google doc spreadsheet. But the one thing that was missing was the serendipitous chatting that happens between/during/after talks.
Other versions of this were using an open-source tool called Jitsi. While this works great, it just doesn’t scale well beyond about 30 people.
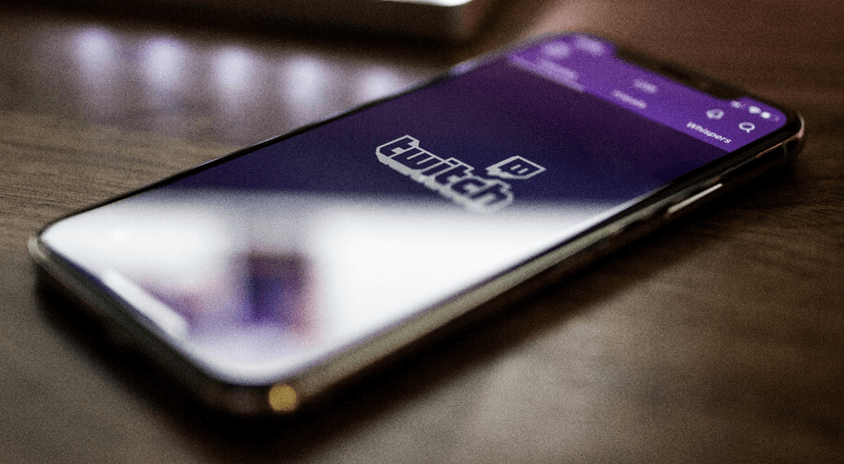
Twitch.tv: or even YouTube:
Twitch is a gaming platform where you can watch many streamers play games. But between Twitch and YouTube they have more recently moved to hosting conferences and it has worked quite well. It has chat functions and is very light in using computer resources. But again, it’s missing those serendipitous chats!
Last but not least:
Sae Ra’s choice! https://venueless.org/en/
It would be remiss if I didn’t bring up an open-source friendly alternative 😊. Venueless is part of a 3-part software package. Venueless is primarily text based however it has 4 key pieces. Sponsor exhibitor spaces, text-based chat rooms, streaming spaces, and video/audio conferencing. What makes this unique is that it’s completely stripped back and a low resource intensive solution. There are no bells and whistles, it’s essentially text-based chat rooms with some video capabilities.
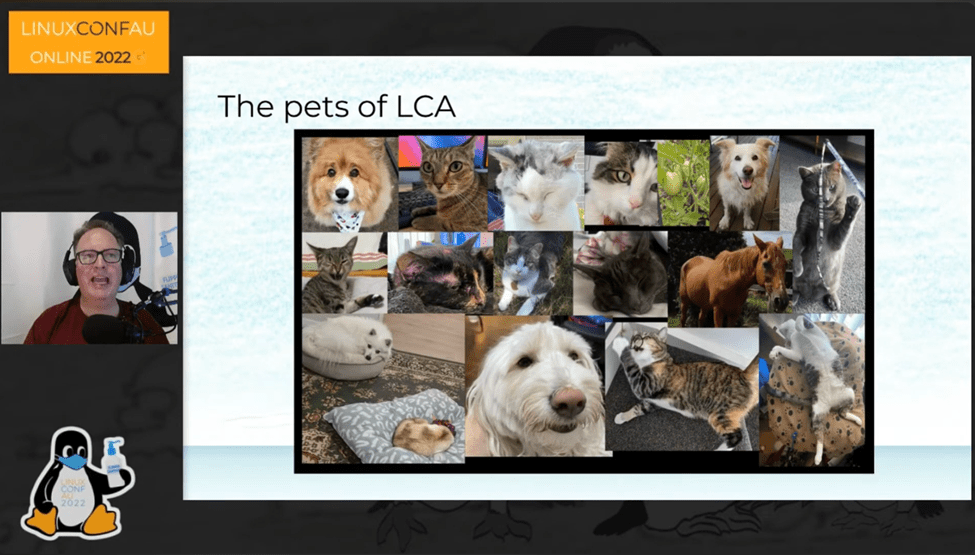
At Linux Australia we used the video/audio conferencing for side birds-of-a-feather sessions, product demonstrations, user group meetings, and we even had our conference dinner in there! One of our speakers wrote their perspective on the event. Running that specific conference gave me all the joy that I would have got in bringing something to people face to face.
One thing to note, is that audio quality is a must. If you are watching a video stream all day, you can forgive video quality because you can look away, but audio quality we aren’t so forgiving about. For some handy tips please go and see this document that we created for linux.conf.au 2021.
After all of this I think it will just go back to the basics: Speakers, Delegates, and Venue. You don’t need the bells and whistles to run a successful conference. Keeping it simple will make your life easier and will make the delegates feel more included.